Vol. 36, No. 1 (2022)
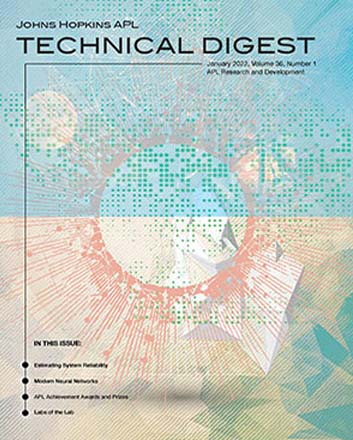
APL Research and Development
Research and development issues highlight APL's diverse contributions to critical national challenges. This issue begins with an overview and examples of a novel and practical method for estimating the reliability of a complex system. The second article, based on a chapter in the award-winning textbook Advanced Signal Processing: A Concise Guide published by McGraw Hill Professional (2020), explores and provides insight into modern neural network concepts and applications. The issue concludes with two special features: a summary of APL's Achievement Awards for work in 2019 and 2020 and a look at a few of the labs of the Lab.
In This Issue
Maximum Likelihood Reliability Estimation from Subsystem and Full-System Tests: Method Overview and Illustrative Examples
This article provides an overview and examples of a novel and practical method for estimating the reliability of a complex system, with confidence regions, based on a combination of full-system and subsystem (and/or component or other) tests. It is assumed that the system is composed of multiple processes, where the subsystems may be arranged in series, parallel, combination series/parallel, or other mode. Maximum likelihood estimation (MLE) is used to estimate the overall system reliability based on the fusion of system and subsystem test data. The method is illustrated on two real-world systems: an aircraft-missile system and a highly reliable low-pressure coolant injection system in a commercial nuclear-power reactor. The examples demonstrate the following properties of the method: (1) Increasing the number of full-system tests improves the confidence in the full-system reliability estimate. (2) Increasing the number of tests of one subsystem stabilizes the subsystem reliability estimate. (3) The likelihood function and optimization constraints can readily be modified to handle systems consisting of repeated components in a mixed series/parallel configuration. (4) A normal distribution approximation for computing confidence intervals is not always appropriate, especially for highly reliable systems. (5) Performing a mixture of full-system and subsystem tests is important when the model that relates the subsystem reliability to the full-system reliability is uncertain.
Modern Neural Networks
Deep neural networks have been tremendously successful in many areas from speech and image recognition to genomics. This article explores and provides insight into modern neural network concepts and applications; it is based on a chapter in the textbook Advanced Signal Processing: A Concise Guide published by McGraw Hill Professional in August 2020.
Special Features
APL Achievement Awards and Prizes: The Lab’s Top Inventions, Discoveries, and Accomplishments in 2019 and 2020
Every year, the Johns Hopkins University Applied Physics Laboratory (APL) honors the accomplishments of its staff members with an awards program. At its inception more than three decades ago, the program recognized staff members’ exceptional contributions to the scientific community via publication. Today’s program continues to recognize outstanding publications but has grown to include awards and prizes celebrating extraordinary achievements in both sponsored programs and internal research and development, efforts that exemplify APL’s focus on transformative innovations, and, most recently, significant contributions that promote a positive, diverse, and inclusive culture at the Laboratory. In 2020, the program underwent yet another change as the Lab pivoted from the formal in-person ceremony on APL’s campus in Laurel, Maryland, to a safe and fun virtual format during the COVID-19 pandemic. This article details the awards presented for achievements in 2019 and 2020.
Labs of the Lab
The Johns Hopkins University Applied Physics Laboratory (APL) creates technologies and innovations that serve national priorities and expand the frontiers of science. By combining creativity and technical expertise with a culture of risk-taking—brought together in cutting-edge collaboration spaces, labs, and test facilities across its campuses—APL’s researchers tackle increasingly difficult challenges with impacts across multiple domains. This article, the first in a recurring series, highlights just a few of the specialized laboratories enabling APL staff members’ critical contributions to the Lab, its sponsors, and the nation.
Inside Back Cover: DART
DART, NASA’s Double Asteroid Redirection Test, is the world’s first planetary defense test mission. After a successful launch from Vandenberg Space Force Base on November 23, 2021, DART is heading for the small moonlet asteroid Dimorphos, which orbits a larger companion asteroid called Didymos, with plans to intentionally crash into the asteroid to slightly change its orbit. While neither asteroid poses a threat to Earth, DART’s kinetic impact will prove that a spacecraft can autonomously navigate to a target asteroid and intentionally collide with it. Then, using Earth-based telescopes to measure the effects of the impact on the asteroid system, the mission will enhance modeling and predictive capabilities to help us better prepare for an actual asteroid threat should one ever be discovered. APL manages the DART mission for NASA’s Planetary Defense Coordination Office as a project of the agency’s Planetary Missions Program Office. NASA provides support for the mission from several centers, including the Jet Propulsion Laboratory in Southern California, Goddard Space Flight Center in Greenbelt, Maryland, Johnson Space Center in Houston, Glenn Research Center in Cleveland, and Langley Research Center in Hampton, Virginia.