News
Scientists Fuse Simulations and Machine Learning to Accelerate Novel Additively Manufactured Materials
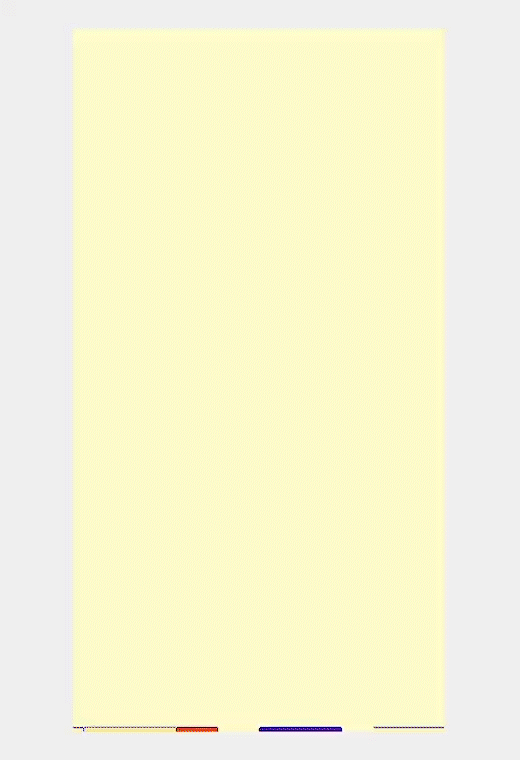
Researchers at the Johns Hopkins Applied Physics Laboratory (APL) in Laurel, Maryland, have demonstrated a novel approach for applying machine learning to predict microstructures produced by a widely used additive manufacturing technique.
Their approach promises to dramatically reduce the time spent and cost of developing materials with tailored physical properties and will soon be implemented on a NASA-funded effort focused on creation of a digital twin.
“We anticipate that this new approach will be extremely impactful in helping design and understand material formation during additive manufacturing processes, and this fits into our overarching strategy focused on accelerating materials development for national security,” said Morgan Trexler, who manages APL’s Science of Extreme and Multifunctional Materials program in the Research and Exploratory Development Mission Area.
The modeling approach focuses on laser powder bed fusion (LPBF), in which layers of metal powder are fused to create 3D objects with a high-powered laser. The process can produce strong, dense metal parts in complex geometries. However, there are many possible variations in processing conditions, resulting from the metal powder characteristics, laser settings and the interactions between these. As a result, the properties of the final materials can vary widely. The key to making LPBF successful, then, is the ability to predict the microstructure of the printed component before printing it, which in turn can be used to predict the mechanical properties of the material and the performance of the fabricated component.
Led by Li Ma, an APL senior staff engineer whose specialties include integrated computational materials modeling for additive manufacturing, researchers developed a technique to predict the microstructure formed during a single laser pass over a certain volume of powder via physics-based computational modeling and simulation.
Specifically, the team used a computational fluid dynamics (CFD) model to quantify changes in temperature and cooling rates during the printing process. Thermal gradient and cooling rate from CFD were used as input into another model that predicts microstructural formation, including grain structure and phase formation. In the future, it will be possible to use these simulations to predict the microstructures of fully manufactured components.
Ali Ramazani, then a senior researcher on the team, performed the detailed modeling work that underpinned this approach, developing the phase field microstructural formation model with the integration of CFD model results. This helped to ensure the accuracy and validity of the simulations and lay the foundation for the development of a machine learning model that followed.
It was a monumental achievement — but it didn’t go quite far enough. Manufacturing a single component entails millions of laser passes. Simulating each small region of that component’s microstructure would take hours of high-performance computing time, severely diminishing the practical advantages of using simulations in the first place.
So, team member Hudson Liu, an APL Student Program to Inspire, Relate, and Enrich (ASPIRE) intern and high school student at Gilman School in Baltimore, developed the final puzzle piece: a machine learning model that would greatly reduce the need to run costly simulations.
Liu combined several existing machine learning model types to create what the team refers to as a diffusion probabilistic field model. Somewhat like the familiar Stable Diffusion platform that creates images from text, Liu’s model generates images based on two key LPBF parameters: the cooling rate and the temperature gradient, which quantifies how temperature changes over distance, particularly from the point of laser impact and the surrounding solid metal.
The model was trained on data from more than 400 simulations conducted at APL, with the microstructure model validated against experimental microscopy measurements of the LPBF material provided by Maureen Williams and Lyle Levine at the National Institute of Standards and Technology in Gaithersburg, Maryland.
The result is a model that can effectively approximate simulations with enough accuracy to dramatically accelerate the process of developing additively manufactured materials using LPBF.
“The key benefit of using a model is its speed. Our model can approximate in seconds or minutes what would take hours in a simulation,” Liu said. “This allows researchers to quickly explore a wide range of parameters and at much lower cost.”
Related Work
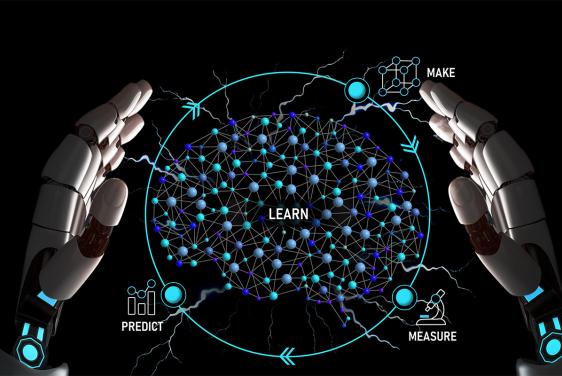