Press Release
Johns Hopkins APL Provides Autonomous Collision Avoidance Technology for Fixed-Wing UAVs
In a first-of-its-kind achievement, researchers at the Johns Hopkins Applied Physics Laboratory (APL) in Laurel, Maryland, have given aerobatic fixed-wing unmanned aerial vehicles (UAVs) the ability to autonomously navigate complex environments using onboard sensing. Central to this significant capability advancement has been APL’s work to equip fixed-wing UAVs with vision-based collision avoidance.
Using an onboard depth camera and a mapping capability called NanoMap, the researchers created a predictive control algorithm that allows the UAV to identify and avoid obstacles. Equipped with this, an operator can safely guide the UAV through extremely constrained spaces — even at high speeds of up to 20 miles per hour.
Success relies on generating both a real-time flight trajectory and “history of depth” images. A stereo camera enables triangulated data gathering of the UAV’s surroundings, giving the vehicle a sense of depth perception, and the control algorithm uses those depth measurements to plan aerobatic maneuvers and avoid potential collisions in real time.
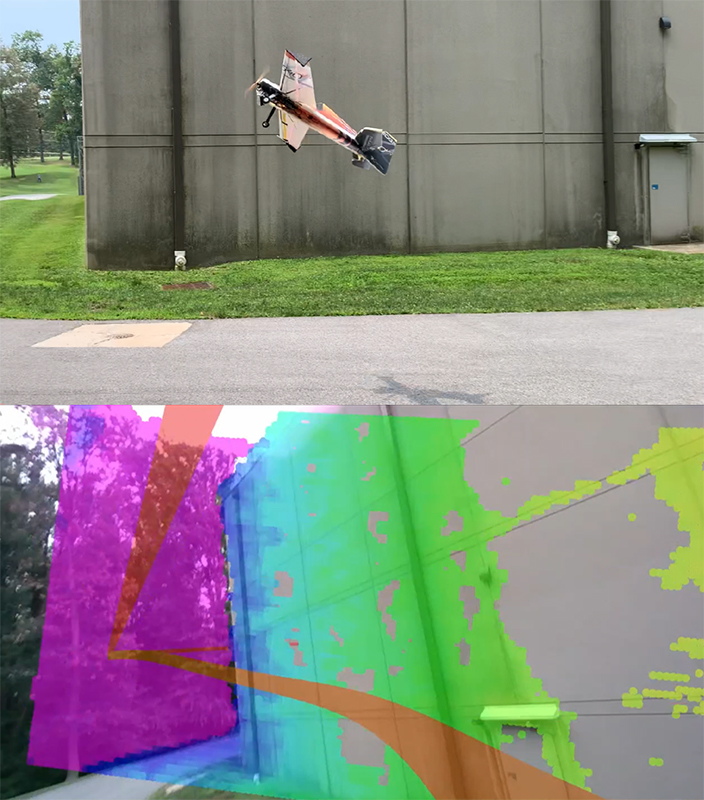
Credit: Johns Hopkins APL
The onboard vision not only allows the UAVs to respond to new or changing environments — freeing their reliance on existing static maps — but also provides important intelligence information.
“If we’re flying in an environment, can we actually do something useful in terms of object detection?” posited Joseph Moore, a robotics and control engineer at APL. “Because one of the things you want to be able to do when you’re flying at high speeds through these environments is detect threats, vehicles or other objects of interest. That’s what sponsors are using our vehicle for — very fast intelligence gathering.”
Moore and his colleagues, which also included APL robotics research engineer Adam Polevoy, published and shared this work at the IEEE International Conference on Robotics and Automation in May 2022.
For Moore and Polevoy, APL’s vision-based collision avoidance represents the culmination of their participation in the Defense Advanced Research Projects Agency (DARPA) OFFensive Swarm-Enabled Tactics (OFFSET) program. In Sprint 5, the fifth and final phase of the program, APL built on Sprint 4 efforts to integrate the Laboratory-developed swarm-enabling capabilities across multiple autonomously coordinating UAVs. During a capstone field exercise in November 2021, APL demonstrated autonomous fixed-wing navigation through narrow urban corridors, all while providing intelligence back to the swarm operator.
In addition to vision-based collision avoidance, APL researchers have designed dynamically actuated gimballed propellers that enable near-vertical takeoffs for fixed-wing UAVs, similar to the takeoffs of their quad-rotor counterparts. When initiated by an operator, the fixed-wing UAV can first elevate into a hover before smoothly transitioning to forward flight. With this capability, the UAV no longer requires considerable space for a cleared runway or a manual launch (in which it is thrown into the air by hand).
“A lot of times, vertical takeoffs and landings are very slow,” explained Moore. “One of the things that we’re able to leverage is the dynamic nature of our takeoff. We don’t have a lot of control authority and roll during takeoff, but because we do it so quickly and get up to speed so quickly, we can dynamically get through that instability phase and into a climb phase.”
Taken together, the autonomous vision-based collision avoidance and near-vertical takeoff allow fixed-wing UAVs to operate in tight places, including those crowded with other coordinating UAVs, ultimately enabling the fixed-wing UAVs to more effectively participate in multi-plane operations and missions.
Through the OFFSET program, participating defense contractors Northrop Grumman and Raytheon now have the opportunity to integrate the APL-developed capabilities across their swarm architecture. The collaboration between DARPA, APL and industry is advancing the operation of heterogeneous robot swarms in urban environments.
Polevoy, who worked on integrating the NanoMap capability and improving the obstacle-avoidance algorithm, looks forward to making further progress. “This work was really exciting because it marked a step forward in our ability to control complex dynamical systems in unknown environments,” he said. “Moving forward, we hope to develop controllers that are better able to reason about dynamics and perception uncertainty.”