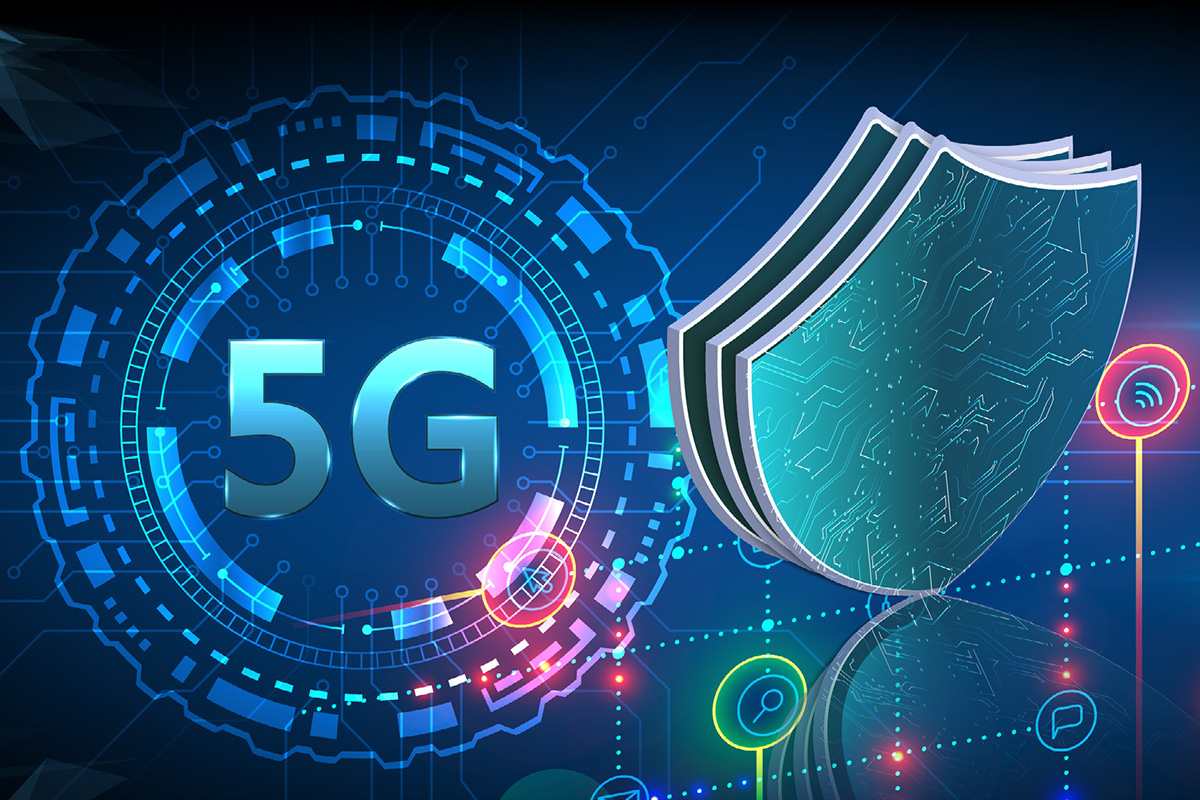
Wireless Cyber Capabilities
Tactical Advantage Through Wireless Protocol Experience
We provide the U.S. military and intelligence communities with operational advantages by leveraging machine learning, software-defined radios, and protocol reverse engineering to develop advanced capabilities for research and operational prototypes. Our engineers apply cutting-edge research to some of our government’s most critical challenges.
We seek to give our nation a significant advantage by controlling the wireless communications environment. We understand sponsor needs, research new technologies, prototype systems, and transition solutions to tactical operations.
Related Publications
Wireless Coexistence: Standards, Challenges, and Intelligent Solutions
Daniel Chew, Andrew L. Adams, and Jason Uher
The Wireless Internet of Things: A Guide to the Lower Layers
Daniel Chew
Wireless Networking: Understanding Internetworking Challenges
Jack L. Burbank, Julia Andrusenko, Jared S. Everett, William T. M. Kasch
Sidelink Mode 2 Operations in Unlicensed Bands: Design Challenges and Potential Approaches
Vijitha Weerackody, Sumit Roy, Kent Benson, and Eric Yang
2023 IEEE Military Communications Conference (MILCOM)
Theoretical Estimation of Passive HF Signal from a Meteorite Plasma Trail
Zachary B. Epstein, Yan Li, Dalibor Todorovski, and Bahman Hafizi
2025 USNC-URSI NRSM
Adversarial Attacks on Deep-Learning RF Classification in Spectrum Monitoring with Imperfect Bandwidth Estimation
Daniel Chew, Daniel Barcklow, Chris Baumgart, and A. Brinton Cooper
2022 IEEE Wireless Communications and Networking Conference (WCNC)
Spectrum Sensing in Interference and Noise Using Deep Learning
Daniel Chew and A. Brinton Cooper
2020 54th Annual Conference on Information Sciences and Systems (CISS)
Cognitive Overlay for Inter-system Cellular Dynamic Spectrum Sharing in the Downlink
Jared S. Everett and Brian L. Mark
2021 IEEE International Conference on Communications Workshops (ICC Workshops)
Persistent Weak Interferer Detection for WiFi Networks: A Deep Learning Based Approach
Andrew Adams, Richard F. Obrecht, Miller Wilt, et al.
arXiv:2205.11360
PHY-NET: A Channel Autoencoder Approach for Wireless PHY Layer Design
Stephan Frisbie, Arun Reddy, Daniel Barcklow, and Stephen Sweeney
2022 IEEE International Conference on Communications (ICC)
AI-Enabled Jammer Deception Using Decoy Packets
Stephan Frisbie and Mohamed Younis
2022 IEEE Global Communications Conference (GLOBECOM)
Data-Driven Wireless Anomaly Detection Using Spectral Features
Stephan Frisbie and Mohamed Younis
2022 IEEE Military Communications Conference (MILCOM)
A Deep Learning Framework for Distributed Channel Selection in a Congested Uncooperative Spectrum
Stephan D. Frisbie and Mohamed Younis
2021 IEEE Global Communications Conference (GLOBECOM)